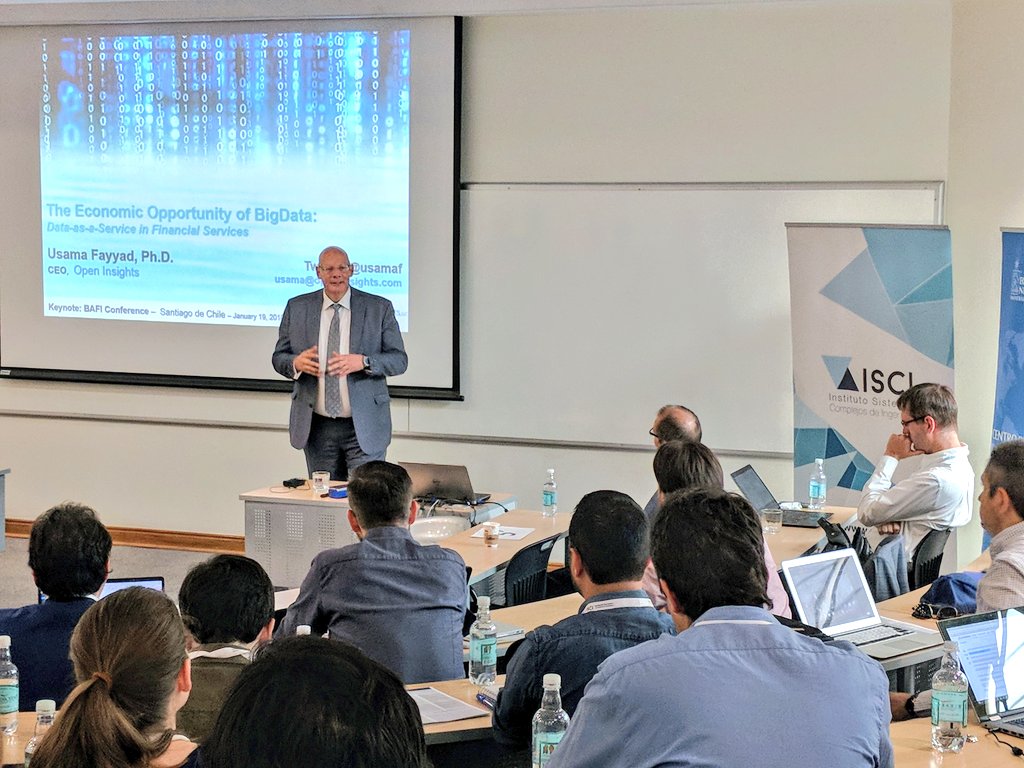
Presentation
The aim of the BAFI 2018 conference is to bring together researchers and developers from data science and related areas with practitioners and consultants applying the respective techniques in different business-related domains. Our goal is to stimulate an academic exchange of recent developments as well as to encourage the mutual influence between academics and practitioners.
At BAFI 2018, the focus will be on methodological developments aimed at uncovering information contained in large data sets, as well as on business applications in various sectors, among them finance, retail, and telecommunications.
Important Dates & General Information
- August 31, 2017: Extended deadline for submission of extended abstracts
- September 10, 2017: Accept/reject decision
- November 15, 2017: Deadline for early registration
- January 17-19, 2018: BAFI 2018
- Conference venue: Santiago, Chile
- Proceedings ISSN 0719-8981
- For information regarding BAFI 2018, please contact info@bafi.cl
Speakers
Gianluca Bontempi 
Gianluca Bontempi is Full Professor in the Computer Science Department at the Université Libre de Bruxelles (ULB), Brussels, Belgium, founder and co-head of the ULB Machine Learning Group. His main research interests are big data mining, machine learning, bioinformatics, causal inference, predictive modelling and their application to complex tasks in engineering (forecasting, fraud detection) and life science. He was Marie Curie fellow researcher, he was awarded in two international data analysis competitions and he took part to many research projects in collaboration with universities and private companies all over Europe. In 2013-17 he has been the Director of the ULB/VUB Interuniversity Institute of Bioinformatics in Brussels. He is author of more than 200 scientific publications, member of the scientific advisory board of Chist-ERA and IEEE Senior Member. He is also co-author of several open-source software packages for bioinformatics, data mining and prediction.
“Machine Learning for Predicting in a Big Data World”
The increasing availability of massive amounts of data and the need of performing accurate forecasting of future behavior in several scientific and applied domains demands the definition of robust and efficient techniques able to infer from observations the stochastic dependency between past and future. The forecasting domain has been influenced, from the 1960s on, by linear statistical methods such as ARIMA models. More recently, machine learning models have drawn attention and have established themselves as serious contenders to classical statistical models in the forecasting community.
See more about Prof. Bontempi here.
Usama Fayyad
CEO Open Insights / www.open-insights.com
He reactivated Open Insights, after leaving Barclays in London. He is also Interim CTO for Stella.AI a Mountain View, CA VC-Funded startup in AI for recruitment. He is acting as Chief Operations & Technology Officer for MTN’s new division: MTN2.0 aiming to extend Africa’s largest telco into new revenue streams beyond Voice & Data.
See more about Mr. Fayyad here.
Peter Flach
Professor of Artificial Intelligence
Intelligent Systems Laboratory, Department of Computer Science
University of Bristol, UK
“The value of evaluation: towards trustworthy machine learning”
Machine learning, broadly defined as data-driven technology to enhance human decision making, is already in widespread use and will soon be ubiquitous and indispensable in all areas of human endeavour. Data is collected routinely in all areas of significant societal relevance including law, policy, national security, education and healthcare, and machine learning informs decision making by detecting patterns in the data. Achieving transparency, robustness and trustworthiness of these machine learning applications is hence of paramount importance, and evaluation procedures and metrics play a key role in this.
In this talk I will review current issues in theory and practice of evaluating predictive machine learning models. Many issues arise from a limited appreciation of the importance of the scale on which metrics are expressed. I will discuss why it is OK to use the arithmetic average for aggregating accuracies achieved over different test sets but not for aggregating F-scores. I will also discuss why it is OK to use logistic scaling to calibrate the scores of a support vector machine but not to calibrate naive Bayes. More generally, I will discuss the need for a dedicated measurement theory for machine learning that would use latent-variable models such as item-response theory from psychometrics in order to estimate latent skills and capabilities from observable traits.
See more about Prof. Flach here.
Francisco Herrera
Head of Research Group SCI2S
Soft Computing and Intelligent Information Systems
Universidad de Granada, Spain
“A tour on Imbalanced big data classification and applications”
Big Data applications are emerging during the last years, and researchers from many disciplines are aware of the high advantages related to the knowledge extraction from this type of problem.
The topic of imbalanced classification has gathered a wide attention of researchers during the last several years. It occurs when the classes represented in a problem show a skewed distribution, i.e., there is a minority (or positive) class, and a majority (or negative) one. This case study may be due to rarity of occurrence of a given concept, or even because of some restrictions during the gathering of data for a particular class. In this sense, class imbalance is ubiquitous and prevalent in several applications. The emergence of Big Data brings new problems and challenges for the class imbalance problem.
In this lecture we focus on learning from imbalanced data problems in the context of Big Data, especially when faced with the challenge of Volume. We will analyze the strengths and weaknesses of various MapReduce-based algorithms that address imbalanced data. We will present the current approaches presenting real cases of study and applications, and some research challenges.
See more about Prof. Herrera here.
Enrique Herrera-Viedma
Enrique Herrera-Viedma is Professor in Computer Science and A.I in University of Granada and currently the new Vice-President for Research and Knowlegde Transfer. His current research interests include group decision making, consensus models, linguistic modeling, aggregation of information, information retrieval, bibliometrics, digital libraries, web quality evaluation, recommender systems, and social media. In these topics he has published more than 200 papers in ISI journals and coordinated more than 20 research projects. Dr. Herrera-Viedma is member of the gobernment board of the IEEE SMC Society and an Associate Editor of international journals such as the IEEE Trans. On Syst. Man, and Cyb.: Systems, Knowledge Based Systems, Soft Computing, Fuzzy Optimization and Decision Making, Applied Soft Computing, Journal of Intelligent and Fuzzy Systems, and Information Sciences.
“Bibliometric Tools for Discovering Information in Science”
In bibliometrics, there are two main procedures to explore a research field: performance analysis and science mapping.Performance analysis aims at evaluating groups of scientific actors (countries, universities, departments, researchers) and the impact of their activity on the basis of bibliographic data. Science mapping aims at displaying the structural and dynamic aspects of scientific research, delimiting a research field, and quantifying and visualizing the detected subfields by means of co-word analysis or documents co-citation analysis. In this talk we present two bibliometric tools that we have developed in our research laboratory SECABA: H-Classics to develop performance analysis by based on Highly Cited Papers and SciMAT to develop science mapping guided by performance bibliometric indicators.
See more information about Prof. Herrera-Viedma here.
Witold Pedrycz
Professor and Canada Research Chair IEEE Fellow
Professional Engineer Department of Electrical and Computer, University of Alberta, Canada
“Linkage Discovery: Bidirectional and Multidirectional Associative MemoriesIn Data Analysis“
Associative memories are representative examples of associative structures, which have been studied intensively in the literature and have resulted in a plethora of applications in areas of control, classification, and data analysis. The underlying idea is to realize associative mapping so that the recall processes (both one-directional and bidirectional) are characterized by a minimal recall error.
We carefully revisit and augment the concept of associative memories by proposing some new design directions. We focus on the essence of structural dependencies in the data and make the corresponding associative mappings spanned over a related collection of landmarks (prototypes). We show that a construction of such landmarks is supported by mechanisms of collaborative fuzzy clustering. A logic-based characterization of the developed associations established in the framework of relational computing is discussed as well.
Structural augmentations of the discussed architectures to multisource and multi-directional memories involving associative mappings among various data spaces are proposed and their design is discussed.
Furthermore we generalize associative mappings into their granular counterparts in which the originally formed numeric prototypes are made granular so that the quality of the associative recall can be quantified. Several scenarios of allocation of information granularity aimed at the optimization of the characteristics of recalled results (information granules) quantified in terms of coverage and specificity criteria are proposed.
See more about Prof. Pedrycz here.
Dominik Ślęzak
Institute of Informatics, University of Warsaw, Poland
See more about Prof. Ślęzak here.
Download Prof. Ślęzak short CV here.
Ronald Yager
Professor of Informarion Systems and Director of the Machine Intelligence Institute at Iona College.
See more about Prof. Yager here.