DIGITAL WITHOUT DATA LEADS TO BLINDNESS: SOLVING THE LAST MILE IN DELIVERING VALUE FROM DATA
SESSION 15A at the MIT CDOIQ SYMPOSIUM on July 22, 2021
Summary:
AI and data solutions are two of the hottest topics in the digital, business and tech worlds. Covid-19 has accelerated digitalization rapidly, yet there has been little consideration about the essential role data plays. For an organization to succeed in this new digital economy of interactions post-Covid, data has to be turned into an asset. The ability to activate AI is highly dependent on availability of quality, granular, data that is reliably labelled for training. This has proven to be a huge challenge for most organizations. While a lot of attention has been dedicated to solving issues of data at rest and data in motion, little progress has taken place on capturing, extracting, and delivering value from data. Data utilization requires capture and management of the right data (structured and unstructured) and requires solving the last mile in data pipelines, specifically around how to safely, securely and privately use data. Today’s data in use protection practices have largely failed, while also having an unknown impact on AI accuracy, which renders them at best a “check the box” and at worst stripping real value from the enterprise. To make AI work an organization must have the necessary data infrastructure and capabilities to make data an asset, instead of a burden. By doing this, organizations have the opportunity to leverage AI in many new ways, including building chatbots, targeting services to customers, and evaluating product relevance for customers. This session will discuss key takeaways from the last three decades on turning data into an asset, protecting data and avoiding the expensive traps when utilizing data. We cover these points:
• How Data is critical for AI to work – generally speaking the only parts of AI that work in pragmatic settings require high quality data for Machine Learning
• The relevance in data for every company, regardless of size
• Today’s practices to protect data in use
• Moving to a model of protecting data in the compute layer, not the data layer
• Why the requirement for real-time data streaming and analysis is stronger than ever
• Safely creating a data sharing/utilization ecosystem – internal and external
• Case studies in creating data strategies for Fortune 500 companies
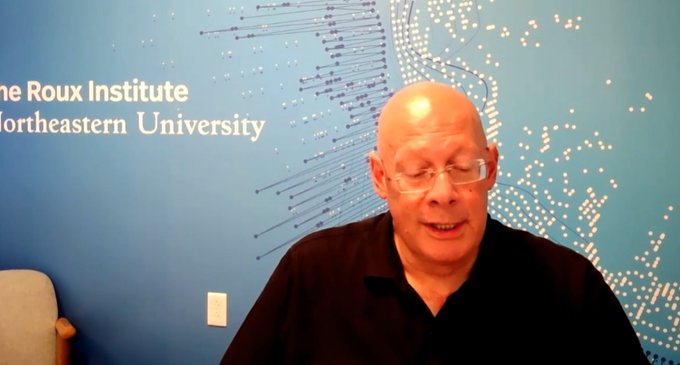