Authors: Ricardo Baeza-Yates, Usama M. Fayyad
Published in: Perspectives on Digital Humanism
Publisher: Springer International Publishing
First Online: 24 November 2021
Abstract
The growing ubiquity of the Internet and the information overload created a new economy at the end of the twentieth century: the economy of attention. While difficult to size, we know that it exceeds proxies such as the global online advertising market which is now over $300 billion with a reach of 60% of the world population. A discussion of the attention economy naturally leads to the data economy and collecting data from large-scale interactions with consumers. We discuss the impact of AI in this setting, particularly of biased data, unfair algorithms, and a user-machine feedback loop tainted by digital manipulation and the cognitive biases of users. The impact includes loss of privacy, unfair digital markets, and many ethical implications that affect society as a whole. The goal is to outline that a new science for understanding, valuing, and responsibly navigating and benefiting from attention and data is much needed.
1 Introduction
We hear frequently of the information overload and its stressful impact on humanity. The growth of networks, digital communications means (most prominently email and now chat), and the plethora of information sources where access is instant and plentiful has resulted in creating a situation where we are often faced with poor, or at best questionable, quality information. This information is often misleading and potentially harmful. But this phenomenon is not limited to those seeking information or connection; the passive entertainment channels are so plentiful that even understanding what is available is a challenge for normal humans, parents, children, educators, and professionals in general.With hundreds of billions of web pages available (Google 2021a, b), how do you keep up? Eric Schmidt, then CEO of Google, was famously quoted as saying that humanity produced more information in 2011 alone than it did in the entire history of civilization, to wit: “There were 5 Exabytes of information created between the dawn of civilization through 2003, but that much information is now created every 2 days” (Huffington Post 2011).
While the accuracy of this information was questionable, it proved to be prophetic of a much more dramatic future that became manifest in the next few years. An article in Forbes (Marr 2018) claimed: “There are 2.5 quintillion bytes of data created each day at our current pace, but that pace is only accelerating with the growth of the Internet of Things (IoT). Over the last two years alone 90% of the data in the world was generated.”A quintillion is 1018 bytes, hence 2.5 Exabytes per day, which about matches the quantity referenced by Eric Schmidt. Whence today, we far exceed the claims made in 2011. Disregarding the fact that most of this “data” should not be counted as data (e.g., copying a video to a distribution point does not constitute data creation in the authors’ opinion), it is unquestionable that recorded information is being generated faster than any time in the history of civilization. Our ability to consume this information as humans is extremely limited and getting more challenged by the day.The real problem is not consuming all this information, as most of it is likely to be of little value. The [now chronic] problem is one of focusing the attention on the right information. We can see this problem even in the world of science or academic publications. The ability to keep up with the quantity of publications in any field has become an impossible task for any particular researcher. Yet the growth continues. Finding the value in the right context is now much harder. Reliance on curated sources—such as journals and conferences—is no longer sufficient. Authors have many more outlets to publish papers, including sharing openly on the Web. While this appears to be a great enabler, it creates a serious problem in determining what is “trusted information.” The problem is compounded by the fact that anyone can write a “news-like” article and cite these various sources. Whether real or “fake” news, these articles have a welcoming environment in social media that enables rapid spread across the Web.So how do we deal with this growing volume of available information? While search engines have been a reasonable approach to cope with casual usage, we are far from having truly powerful search technology. Understanding the meaning and context of a short query is difficult. Search engines that have a good understanding of content and are not as reliant on statistical keyword search are not yet practical. As we explain in the next section, there are many areas of the new “attention economy” that still need new solutions that are practical. We believe that all the demand points to the need for search engines that understand semantics, context, intent, and structure of the domain being searched: from health records, to legal documents, to scientific papers, to drug discovery, to even understanding the reliability of the sources. Finally, with social media driving crowdsourcing of user-generated content, a growing importance is played by monitoring and reliably assessing the type of content being shared and whether it is appropriate by social, legal, privacy, and safety criteria and policy. These are all wide open areas needing new research.
2 The Attention Economy
Since the scarce resource is attention, an economy around getting to people’s attention has rapidly grown. One only needs to examine the growth of digital advertising over the last decade or two to see economic evidence of the market value of attention.Digital advertising on the Internet has grown from an estimated $37B market in 2007 to being well over $360B market in 2020 (Statista 2021) as shown in Fig. 1 trending the growth rate and breakdown of online advertising into the three major categories: search advertising, display advertising (graphical ads), and classified listings.
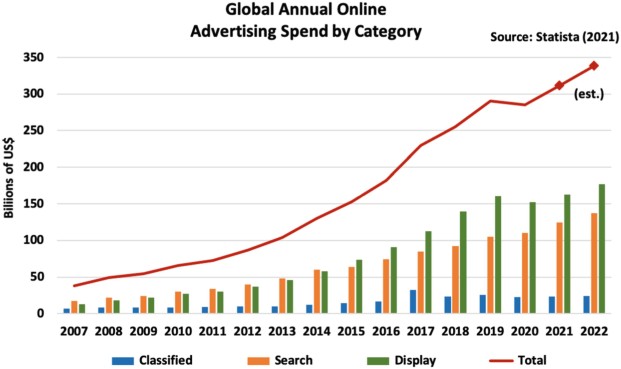
This is a good proxy as it actually underestimates the true value since many advertising markets are not yet matured. However, it is an indication of the growth of time spent by audiences online. The majority of the ad spend is on advertising on mobile devices as this medium drove most of the online growth in the last 7 years (e.g., US ad numbers (Forbes 2019)).We use web content consumption as a proxy. The problems are actually deeper in areas that have not quite been valued economically yet, but are playing a bigger and more fundamental role in our digital lives, both as individuals and organizations/companies.Underlying the growth of the attention economy is the data economy and what that data is worth to organizations and individuals. This problem is different from content on the Web and is where we have yet to see most of the growth and interesting innovations. Advertising and “hacked data” on the dark web provide underestimates of this economy. From storing the data, to maintaining it, to creating cloud services to enable secure access and management, this is likely to become the big economy of the future.Many attempts at sizing the data economy have faced many challenges. Li (2020) found that data use enables firms to derive firm-specific knowledge, which can be measured by their organizational capital, the accumulated information, or know-how of the firm (Prescott and Visscher 1980); the more the data, the greater the potential derived firm-specific knowledge. They estimated the organizational capital for each of top seven global online platform companies, Apple, Amazon, Microsoft, Google, Facebook, Alibaba, and Tencent, and compared their combined organizational capital with the global data flow during the same period of time. This provides evidence that large online platform companies have been aggressively investing capital in order to tap the economic opportunities afforded by explosive global data growth, which leads to some estimates of the size of the data economy.No accepted methodology to measure the value of the market for data exists currently. Apart from the significant conceptual challenges, the biggest hurdle is the dearth of market prices from exchange; most data are collected for firms’ own use. Firms do not release information relating to transactions in data, such as the private exchange or the sharing of data that occurs among China’s big tech companies. Li et al. (2019) examine Amazon’s data-driven business model from the perspectives of data flow, value creation for consumers, value creation for third parties, and monetization. Amazon uses collected consumer data and, through recommendations, insights, and third-party services, creates new products and services for its users, for example, personalized subscription services and recommendations for all products and services sold on its marketplace. “In 2019, Amazon had more than 100 million Prime subscribers in the U.S., a half of U.S. households, with the revenue from the annual membership fees estimated at over US$9 billion” (Tzuo and Weisert 2018). By taking advantage of insights on interactions and transactions, Amazon can capture much of the social value of the data they have accumulated. This is why any attempt at sizing the data economy can be challenging: the plethora of refinement and reuse forms a huge and poorly understood, yet rapidly growing, space.What role does AI play in helping us deal with these information overload problems? We believe that since technology was the big enabler of the information overload, technology will also hold the keys to attempt to tame it. Because what we seek is an approach to filter information and focus attention, we naturally gravitate to approaches that are based on what is typically referred to as AI. The reason for this is that generating and collecting data and information does not necessarily require “intelligence.” But the inverse problem: determining meaning and relevance does require it.In order to filter, personalize, and meaningfully extract information or “reduce” it, there needs to be an understanding of the target consumer, the intent of the consumer, and the semantics of the content. Such technologies typically require either the design and construction of intelligent algorithms that code domain knowledge or employing machine learning to infer relevance from positive, negative, and relevant feedback data. We discuss this approach further in Sect. 3.The main theme is that AI is necessary and generally unavoidable in solving this inverse problem. This brings in complications in terms of an ability to correctly address the problem, to effectively gather feedback efficiently, and, assuming the algorithms work, to issues of algorithmic bias and fairness that we shall discuss in Sect. 4.
3 The User-Machine Feedback Loop
Much of the attention economy is constructed upon the interaction feedback loop of users and systems. The components of such a loop are discussed here, and in the next section we detail the biases that poison them.The overall setup of this problem as an AI problem is totally analogous to a search engine operation. Web search requires four major activities:1.Crawling: web search engines employ an understanding of websites and content to decide what information to crawl, how frequently, and where to look for key information. 2.Content modeling: requires modeling concepts and equivalences, stemming, canonization (e.g., understanding what phrases are equivalent in semantics), and reducing documents to a normalized representation (e.g., bag of words, etc.). 3.Indexing and retrieval: figuring out how to look up matches and how to rank results. 4.Relevance feedback: utilizing machine learning (MLR) to optimize the matching and the ranking based on user feedback: either directly or by leveraging information like click-through rates, etc. Each of these steps above requires some form of AI. The problem of capturing relevance and taming the information overload in general requires us to solve the equivalent components regardless of the domain of application: be it accessing a corpus of scientific applications, tracking social media content, determining what entertainment content is interesting, or retrieving and organizing healthcare information.We note that this framework has the human-in-the-loop component for capturing the scarce resource accurately: is this content interesting for the target task? This natural loop is an example of leveraging AI either through direct feedback or through the construction of reliably labeled training data. The next three elements need serious consideration as we consider approaches to “human-in-the-loop” solutions.
3.1 Users and Personal Data
The first key element is the digital identity as a user. A digital identity can range from anonymous to a real personal identity. However, in practice, you are never completely anonymous as you can be associated with an IP address, a device identifier, or/and a browser cookie. If you are authenticated in a system, you will have more identification data associated with you, but part of it might be fake by choice.The second element is data protection, that is, how secure is your personal data stored by the system that is using it. In many countries, this is regulated by law. The most well-known case is the General Data Protection Regulation (European Union 2016) of the European Union. This regulation includes when user consent is needed, proportionality principles for the amount of data that can be requested, how long it can be stored, and how it can be processed and transferred.The third element is privacy, the ability of an individual or group to seclude information about themselves. For this you need at least data protection but if you can also conceal your digital identity, even better. Privacy is a shared attribute in the sense that does not depend on just the individual but the network of relations that a person has. It does not matter how private you are if the people that you know share more information about you. For this reason, some people even argue that privacy is collective (Véliz 2021) although in the United Nations Declaration is an individual human right (United Nations 1948).
3.2 Algorithms
Any software system is built on top of many algorithms. Some key components are the algorithms used to gather and store data about the environment and the users, profile the users to personalize and/or nudge their experience, and monetize their interaction. Tracking and profiling users can be done at an individual level or can be coarser, such as assigning a particular persona to each user. A “persona” is a construction of a realistic exemplar or actual specific user that represents a segment of users having certain preferences or characteristics. Apple, for example, uses differential privacy to protect individual privacy, and in the next release of its mobile operating system, iOS 14, users will decide if they can be tracked or not (O’Flaherty 2021). At the same time, Google is planning to move away from cookies by using FLoCs or Federated Learning of Cohorts (Google 2021b). Nudging implies manipulating the behavior of the user, from suggesting where to start reading to where we place elements that we want the user to click.
3.3 Digital Information Markets
The attention economy has created a specific data economy that refers to tracking and profiling users (as discussed above). For this reason, although talking about television, Serra and Schoolman in 1973 said that “It is the consumer who is consumed, you are the product of T.V.” So, we are the product and the data economy is at the center of digital information markets. Digital information markets are platforms/environments that have different types of incentives that form a market. They include social networks, ecommerce, search engines, media streaming, etc. One characteristic of these markets is that they have popular as well as a long tail of items (e.g., products or movies). Users also differ on engagement, creating another long tail of interaction and hence tracked data. Most digital markets optimize the short-term revenue and hence are not necessarily optimal. All these characteristics shape the system’s feedback loop.
4 Biases
In this section, we cover many relevant biases that exist in software systems, particularly ML-based systems. Bias is a systematic deviation regarding a reference value, so in principle the word is neutral. However, we usually think about bias in a negative way because in the news only negative biases are covered (gender, race, etc.). We can distinguish between statistical bias, product of a measurement; cultural or social bias; and cognitive bias that are particular to every person. We organize them by source, data and algorithms, including the interaction of users with the system.
4.1 Data
This is the main source of bias as data may encode many biases. In Table 1, we show examples of different types of generic data sets crossed with key examples of social bias that might be present, where Econ represents wealth-based discrimination. However, biases might be subtle and not known a priori. They can be explicit or implicit, direct or indirect. In addition, sometimes it is not clear what should be the right reference value or distribution (e.g., gender or age in a given profession).Table 1Example of biases found on different types of data sets
Data set | Gender | Race | Age | Geo | Econ |
---|---|---|---|---|---|
Faces | ✓ | ✓ | ✓ | ✓ | ✓ |
News | ✓ | ✓ | ✓ | ✓ | ✓ |
Resumes | ✓ | ✓ | ✓ | ✓ | ✓ |
Immigration | ✓ | ✓ | ✓ | ✓ | |
Criminality | ✓ | ✓ | ✓ | ||
Recidivism | ✓ | ✓ | ✓ |
One important type of data that encodes many biases is text. In addition to gender or racial bias, text can encode many cultural biases. This can even be seen when it is used to train word embeddings, large dimensional spaces where every word is encoded by a vector. There are examples of gender bias (Caliskan et al. 2017), race bias (Larson et al. 2016), religious bias (Abid et al. 2021), etc., and their impact has many ramifications (Bender et al. 2021).There can be biases also in how we select data. The first one is the sample size. If the sample is too small, we bias the information (say events) in the sample to the most frequent ones. This is very important in Internet data as the probability of an event, say a click, is very small and the standard sample size formula will underestimate the real value. Hence, we need to use adapted formulas to avoid discarding events that we truly want to measure (Baeza-Yates 2015). In addition, in the Internet, event distributions usually follow a power law with a very long constant tail and hence are very skewed. For this type of distribution, the standard sampling algorithm does not generate the same distribution in the sample, particularly in the long tail. Hence, it is important to use a stratified sampling method in the event of interest to capture the correct distribution.
4.2 Algorithms
Bias in algorithms is more complicated. A classic example is recommending labels in a platform that gathers labels from users. The availability of recommendations will incentivize users to put less labels. If the system recommends labels without any seed label, there will be no more new labels, and hence the algorithm cannot learn anything new. In other words, the algorithm itself kills the label folksonomy (ironically helping people reduce data coming from them!).In other cases, the function that the algorithm optimizes, perhaps designed with best intentions, produces new or amplifies existing bias. Examples include race bias amplification in bail prediction (Kleinberg et al. 2018) or delivery time bias in food delivery (Forbes 2021). Part of the problem here is that many times the focus of the designers is on increasing revenue without thinking about the indirect impact of what is being optimized. Moreover, recently, the first indication that programmers can transfer cognitive biases to code was published (Johansen et al. 2020), an example of more subtle indirect bias.The largest source of algorithmic bias is in the interaction with the user. First, we have exposure or presentation bias. Users can only interact with what is presented to them and that is decided by the system. Hence, if the system uses interaction data for, say, personalization, the system is partly shaping that data. Because of the attention economy, the amount of interaction of users also follows a power law, creating an engagement or participation bias (Nielsen 2016; Baeza-Yates and Saez-Trumper 2015).One of the main effects of the interaction feedback loop is popularity bias. That is, popular items get more attention than they deserve with respect to items in the long tail. Other effects depend on cognitive biases of the users such as how they look at the screen, how much they are influenced by the information read, or how often they click or move the mouse. This creates position bias and, in the case of search engines, ranking bias (Baeza-Yates 2018). That is, top ranking positions get more clicks only because they are at the top. To counteract this bias, search engines debias clicks to avoid fooling themselves. In addition, ratings from other users create social pressure bias.
5 Societal Impact
There are several areas of impact on society which are summarized by:1.How the data economy creates a loss of privacy. A loss of privacy that many people are not aware of as they normally do not read the terms of usage. Shoshana Zuboff calls this surveillance capitalism (Zuboff 2019), or surveillance economy, to distinguish it from government surveillance, as it is mainly carried out by large Internet multinationals. Carissa Véliz (2021) argues that “whoever has the most personal data will dominate society.” Hence, privacy is a form of power: if companies control it, the wealthy will rule; while if governments control it, dictatorships will rule. The conclusion is that society can only be free if people keep their power, that is, their data. She goes on augmenting that data is a toxic substance that is poisoning our lives and that economic systems based on the violation of human rights are unacceptable, not only because of ethical concerns but because the “surveillance economy threatens freedom, equality, democracy, autonomy, creativity, and intimacy.” 2.Digital manipulation of people. This goes beyond the digital nudging explained earlier, say to entice you to click an ad without your noticing it. The main example is social media and fake news. This new social age is ruled by what Sinan Aral calls The Hype Machine (Aral 2020), a machine that has disrupted elections, stock markets, and, with the COVID-19 pandemic, also our health. There are many examples of country-wide manipulation from governments such as in Brazil, Myanmar, and the Philippines or from companies such as Cambridge Analytica using Facebook data which affected the 2016 US presidential election. Harari is much more pessimistic as some fake news lasts forever and “as a species, humans prefer power to truth” (Harari 2018). The future danger for him is the combination of AI with neuroscience, the direct manipulation of the brain. In all of the above, AI is the key component to predict which person is more prone to a given nudging and how to perform it. 3.Unfair digital markets (monopolistic behavior and the biased feedback loop previously mentioned). During 2020, the US government started antitrust cases against Facebook (US FTC 2020) and Google (US DoJ 2020). However, the popularity bias also discriminates against the users and items in the tail, creating unfairness and also instability. Better knowledge of the digital market should imply optimal long-term revenue and healthier digital markets, but the current recommendation systems are optimized for short-term revenue. In some sense, the system is inside a large echo chamber that is the union of all the echo chambers of its users (Baeza-Yates 2020). 4.Ethical implications of all the above. That includes discrimination (e.g., persons, products, businesses), phrenology (e.g., prediction of personality traits based in facial biometrics (Wang and Kosinski 2018)), data used without consent (e.g., faces scrapped from the Web used to train facial recognition systems (Raji and Fried 2020)), etc. There are also impacts in specific domains such as government, education, health, justice, etc. Just to exemplify one of them, we analyze the impact on healthcare. While machine-driven and digital health solutions have created a huge opportunity for advancing healthcare, the information overload has also affected our ability to leverage the richness of all the new options: genome sequencing, drug discovery, and drug design vs. the problem of understanding and tracking what this data means to our lives. How the healthcare industry is having trouble dealing with all the data is manifested in these examples:
- Lack of standards created data swamps in electronic health records (EHRs)
- Lack of ability to leverage data for population healthcare studies and identify panels on demand
- Lack of ability to leverage collected data to study drug and treatment impacts systematically
- Inability of healthcare providers to stay on top of the data for an individual
- Lack of individuals in ability to stay on top of data about themselves
6 Conclusions
As the tide of information overload rises, we believe that this makes the traditional long tail problem even harder. The reason for this is that the information coming from the head of the tail, the most common sources of new data or information, is not growing as fast as information coming from the less popular sources that are now enabled to produce more growth. This exacerbates the long tail distribution and makes information retrieval, search, and focus of attention much more difficult.As a final concluding thought, one may wonder if the only way out is through use of AI algorithms. The answer is a qualified yes. There may be better solutions through design, through proper content tagging and classification and modeling as content is generated. But the reality is that for the majority of the time, we are living in a world where we have to react to new content, new threats, new algorithms, and new discovered biases. As such, we will always be left with the need for a scalable approach that has to solve the “inverse design” problem—inferring from observations what is likely happening. This seems to drive “understanding,” especially semantic modeling, to the forefront. And this seems to drive us to look for algorithms to solve such inference problems, and whence AI.There are other related issues that we did not cover. Those include cybersecurity and the growing dark web economy, as well as other emerging technologies that create synergy with AI. The same for the future impact of the proposed regulation for AI in the European Union that was just published (EU 2021).Open Access This chapter is licensed under the terms of the Creative Commons Attribution 4.0 International License (http://creativecommons.org/licenses/by/4.0/), which permits use, sharing, adaptation, distribution and reproduction in any medium or format, as long as you give appropriate credit to the original author(s) and the source, provide a link to the Creative Commons license and indicate if changes were made.The images or other third party material in this chapter are included in the chapter’s Creative Commons license, unless indicated otherwise in a credit line to the material. If material is not included in the chapter’s Creative Commons license and your intended use is not permitted by statutory regulation or exceeds the permitted use, you will need to obtain permission directly from the copyright holder.
View online